Key insights
Closely aligning your data strategy with business objectives can drive organizational transformation and create significant value.
It’s important to achieve short-term successes to demonstrate value quickly, but these should be integrated into a broader long-term strategy to help avoid inefficiencies and promote sustainable growth.
Generative AI can streamline processes and uncover previously hidden insights in data. However, successful adoption of AI requires a robust data foundation.
Data debt, which involves fragmentation, redundancy, and inefficiencies in data storage and access, must be managed strategically.
As technology continues to evolve, the accounting industry must embrace a proactive and strategic approach to data. AI, automation, and advanced analytics offer incredible opportunities, but they depend on a solid foundation of well-managed, high-quality data.
Collaboration and innovation can create a culture of data-driven excellence that propels organizations toward sustained success. This means:
- Data strategy should map directly to business strategy,
- A short-term forecast may need to be used within a longer-term vision,
- Potential debts must be understood and payable over the long term, and
- Relationships need to be built to truly transform an organization from a digital and data perspective.
The importance of a data strategy
The intersection of business and data strategy has become a critical focal point in modern accounting. To enhance data potential, it’s essential to align it with your organization’s overarching business goals. Data strategy should serve as a catalyst for organizational transformation and value creation, driving initiatives that support long-term objectives.
Success lies in deeply understanding business problems and crafting data-driven solutions that prioritize impactful results. When initiating any data project, focus on stakeholder pain points and strategic aspirations. Conversations should not only address current frustrations but also identify opportunities for innovation aligning with your organization’s broader vision.
A robust data strategy begins with posing the right questions, identifying the most pressing business challenges, and harmonizing data initiatives with the overall strategic framework. This approach helps every data effort contribute meaningfully to your organization’s sustained growth and success.
The balancing act: Short-term wins vs. long-term vision
Every organization faces pressure to deliver immediate results. Short-term wins can demonstrate value quickly, but they must be carefully integrated into a broader long-term strategy to avoid creating inefficiencies. The concept of “data debt” — accumulated inefficiencies in data systems — poses a significant challenge. When left unchecked, data debt can hinder future innovation and limit scalability.
A practical approach to this balancing act involves using proofs of concept (POCs) to validate ideas on a smaller scale. These POCs allow teams to learn quickly, iterate, and adjust without committing excessive resources. However, POCs must always be designed with the long-term architecture in mind while building toward sustainable and scalable solutions.
Prioritization is key: Efforts should focus on initiatives delivering the greatest impact relative to the effort required. By overlaying metrics such as business value, stakeholder impact, and resource investment, teams can make informed decisions about where to direct their focus.
The generative AI revolution in accounting
Generative AI is ushering in a new era of innovation, with its potential to revolutionize the accounting industry by streamlining processes and uncovering insights previously hidden in data, historically requiring many hours of highly skilled data workers and AI technologists to uncover.
However, successfully adopting AI requires a robust foundation. Data governance, integration, and quality are critical prerequisites for unlocking AI’s potential. You cannot have an AI-empowered organization at scale without governance.
The process of building AI solutions should be tightly integrated with efforts to address data debt. This involves creating centralized, governed repositories where data is organized and accessible for both immediate and long-term use. By embedding AI into these systems, your organization can align its efforts and reinforce broader strategic goals.
Leadership and transparency in data decision-making
Transparency in data strategy is essential for fostering trust and collaboration. Every data initiative involves competing priorities, and not every request can be fulfilled immediately. To manage this effectively, teams must communicate openly about how decisions are made, the factors influencing those decisions, and the expected outcomes.
Centralized visibility into data projects can help stakeholders understand the bigger picture. For example, maintaining a shared roadmap of initiatives allows business leaders to see how their priorities fit within the broader organizational strategy. Transparency also provides opportunities to gather valuable feedback, enabling teams to adapt and align more effectively.
Leadership in this space requires balancing short-term problem-solving with the discipline to pursue long-term goals. This often means making tough decisions about where to allocate resources and delivering clear, honest communication about the reasoning behind those decisions.
Strategies for managing data debt
One of the biggest hurdles in this journey is addressing data debt. Organizations may possess vast quantities of data locked in disparate systems, created over years of acquisitions, mergers, and operational changes. To move forward, there must be a strategy for harmonizing this data, making it accessible, leveraging it for decision-making, and maintaining it so your asset stays in good working order.
The growing complexity of data environments has made managing data debt a top priority for organizations. Unlike technical debt, which often involves system upgrades, data debt deals with the fragmentation, redundancy, and inefficiencies in how data is stored and accessed. Addressing data debt requires a multifaceted approach balancing immediate needs with long-term transformation.
The key to success is incremental progress. Focus on high-value areas first — those delivering the greatest impact relative to the resources required. For instance, integrating data from key operational systems into centralized platforms can provide immediate benefits while laying the groundwork for future AI-driven initiatives.
Data debt is not insurmountable, but it requires strategic planning. View each project as an opportunity to build toward a unified, governed data environment consistently stored and explainable to all data consumers. Over time, these incremental improvements can compound, creating a system that is not only efficient but also positioned to support advanced capabilities like AI.
Connect
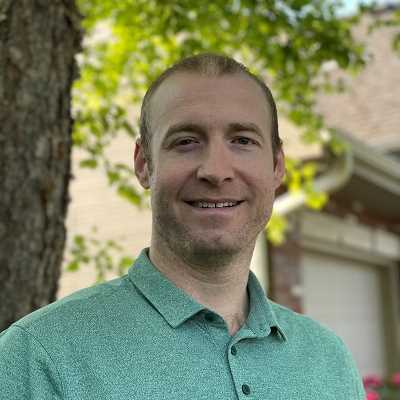
Spencer Lourens
Chief Data Officer