Key insights
Digital transformation can bring significant opportunities for your organization, including the potential of artificial intelligence (AI) and predictive analytics.
AI is rapidly evolving, with advancements in computer vision and large language models, and is becoming more accessible and user-friendly.
While there are challenges to implementing AI in a business context, prioritizing data quality, addressing integration issues, and implementing stringent security measures can help organizations navigate these complexities.
Digital transformation can bring significant opportunities for your organization. It’s an evolution that spans dashboard creation, data estates and warehouses, and the potential of artificial intelligence (AI) and predictive analytics.
Consider how your organization can understand and harness transformative technologies like AI and predictive analytics. Let’s walk through the trends, see how it’s being used in the business world, and review potential challenges.
The rapidly evolving world of AI and predictive analytics
From the early days of explicit instructions to the current era of machine learning and deep learning, AI has made significant strides — and many anticipate those advancements will continue.
AI is not just a passing trend. The growth of the cloud is making storage and computing power more cost-effective and accessible, further fueling the proliferation of AI.
Computer vision
Computer vision enables AI to understand visual information from the world. As a result, AI can detect and interpret objects at an exceptional rate, enhancing its applicability in real-world scenarios ranging from autonomous driving to image recognition and analysis.
Large language models
Large language models are set to play a significant role in shaping the future of AI. Models such as ChatGPT have already showcased their potential in natural language processing.
As large language models continue to evolve, they may offer increasingly sophisticated capabilities, enhancing the functionality of AI and contributing to its broader adoption and utility.
Bringing AI technology to the masses
It’s becoming more common to incorporate AI into existing tools, making AI models more user-friendly and accessible to a wider audience.
This democratization is akin to the evolution of computers, which transitioned from being sequestered in guarded spaces to being handheld devices, enabling everyday individuals to take advantage of the technology. And the more individuals and organizations use AI, the more valuable the technology becomes.
Integrating AI into business processes
AI and predictive analytics are being integrated into organizations that want to move beyond basic data analysis. The technology offers tangible benefits where businesses can save significant time and resources, enabling employees to focus on more critical tasks.
Consider these diverse business use-cases:
- Document automation using computer vision models allows businesses to extract and summarize data to process invoices and contracts. This process also integrates human review to check the accuracy and reliability of the extracted information.
- Large language models are used to generate content, provide email responses, and assist in language translation, enhancing overall operational efficiency.
- A large language model could predict employee turnover with an accuracy of 98.8%, demonstrating the immense potential of AI in data analysis and business decision-making.
- Integrating large language models into data analysis can help interpret and visualize data, as well as generate a comprehensive report to present to leadership.
Interested in learning more?
Watch additional video clips from our Data Analytics: Crawl, Walk, Run — Run webinar.
Navigating the challenges of AI in business
Understanding and implementing AI effectively in a business context is a common challenge. This includes understanding how AI can help, knowing how to implement it, managing data, and keeping up with the fast pace of AI developments.
Data quality concerns
The model is only as good as the underlying data, so data quality is a primary concern. Many organizations struggle with getting their data in a suitable format for AI analysis, and data quality directly impacts the accuracy and reliability of AI models.
Integration issues
As businesses seek to incorporate AI into their existing tools and processes, issues can arise. Integrating AI models into your current infrastructure can be complex and requires thoughtful planning for smooth implementation.
AI security challenges
Data is critical for AI. Prioritize developing robust security measures to safeguard sensitive information and prevent unauthorized access. Carefully address potential AI security risks to maintain the integrity of AI-driven processes.
Ready to go deeper?
Check out the previous webinars in our data analytics series to learn more about digital transformation:
Data Analytics: Crawl, Walk, Run — CRAWL: Effectively Managing Data.
Connect
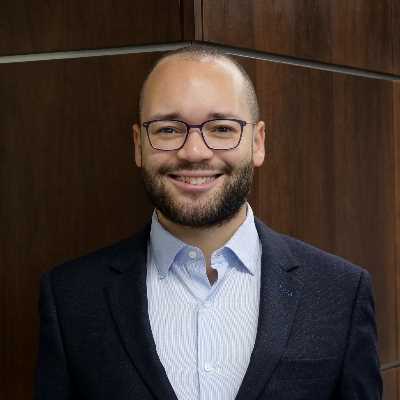
Gregory Chambers
Data Analyst Manager
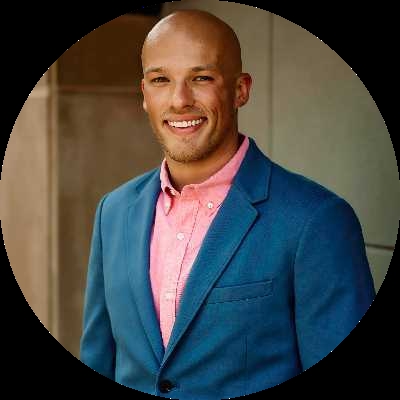
Alexander White
Data Scientist Manager