Key insights
Data management is crucial for business intelligence and strategic decision making.
A data estate includes data assets, storage technology solutions, infrastructure, and processes used to harness and analyze data sources.
Assess your organizational needs and factors like your size, budget, and security requirements to determine which of the multiple data estate options might work best for you.
Your data is an asset. In our increasingly digitized world, data management has become the cornerstone of business intelligence and strategic decision making.
Many organizations start their digital transformation with dashboards. How do you take it to the next level? To capitalize on vast amounts of information, organizations must deploy sophisticated data storage systems.
Read on to explore the critical role of data infrastructure. Learn how the proper architecture can drastically enhance your organization’s analytics capabilities.
Assessing your data estate
Think about your data environment as a data estate — a collection of data assets of all types and from various internal and external sources. It also includes the data storage technology solutions, infrastructure, and processes.
When you bring data into a central place that’s organized and where systems play nicely with each other, you can gain valuable insights and make better business decisions.
Delving into data infrastructure: lakes, warehouses, and marts
As the amount of data increases, data storage systems grow more complex and each one serves a specific purpose.
A data estate can include key structures such as data lakes, data warehouses, and data marts — and they are pivotal to navigating and analyzing diverse data sources.
Data lake
A data lake is a centralized repository storing data from various source systems, such as SQL databases, cloud providers, and social media. It’s designed to be flexible and scalable so organizations can accumulate and retain large volumes of raw data in its native format until needed.
Data warehouse
Like a data lake, information is brought into a data warehouse from multiple systems, but the data warehouse is structured to provide a system-agnostic view of an organization’s data. It allows data to be represented in a standardized format, making it easier to analyze and understand regardless of the source system.
Data mart
This customized database is tailored to meet the analytical needs of a department or project. It’s designed for a specific use case, such as the finance department’s view of the organization.
How do data storage systems work together?
These structures are designed to provide a comprehensive view of the organization, enabling effective performance analysis. Data structures such as data warehouses and data marts are essential for organizing and storing data in a way that makes it accessible and actionable.
But how might you integrate the different options?
- A data lake is the most broad, flexible storage option and many organizations start with a data lake. A data lake could feed a data warehouse.
- The data warehouse is more structured with business rules behind it. It’s intended to give you the facts and some dimensions — an enterprise view of the organization anyone can see. The data warehouse could hold information valuable for finance, operations, sales, and more.
- Your data mart is then designed to provide a subset of the data useful for a particular department or specific project. A data mart could be standalone or built off your data warehouse.
Introducing the data lakehouse model
The concept of a data lakehouse has emerged as a powerful data management resource in modern organizations. A data lakehouse combines the best features of a data lake and a data warehouse, offering a versatile and comprehensive platform for data management.
Just like a data lake, a data warehouse can handle structured, semi-structured, and unstructured data, so it can serve as a unified repository for all types of data. While a data lakehouse is not a traditional database, it can function like one, allowing users to run queries and extract information.
Tapping into machine learning and AI capabilities
The data lakehouse is enhanced for machine learning and AI, making it well-suited for advanced analytics and predictive modeling.
Machine learning has become increasingly important in driving business performance and gaining valuable insights from data. Businesses can uncover patterns and trends, leading to more informed and strategic decision making. Organizations are leveraging the power of machine learning to improve efficiency, enhance customer experiences, and drive innovation.
Industries can also leverage predictive analytics to forecast trends, identify potential risks, and enhance operations. This flexible and efficient environment can help organizations seamlessly transition from raw data ingestion to deriving valuable insights.
Connect
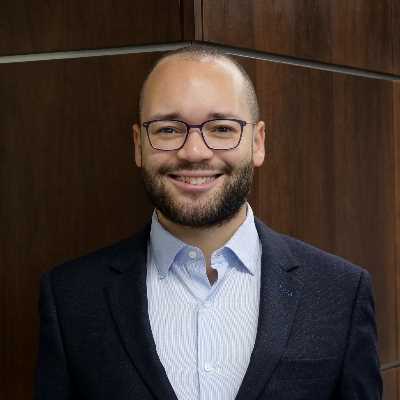
Gregory Chambers
Data Analyst Manager